What is hedging? It’s “a way of controlling or limiting a loss or risk“, isn’t it?
Let’s assume chatGPT has the typical opinion from a commodity market practioner: “Commodity hedging is a risk management strategy used by businesses to protect against price fluctuations in raw materials or goods they rely on by entering into futures contracts or other derivative instruments.“
It seems rather the opposite of speculation. Really? The frontier between both concepts is probably harder to define, if you think twice. In 1967, MIT researcher Paul Cootner said here:
In most circumstances, hedging is really a form of speculation – speculation of the basis. It has all the characteristics of speculation even though it is an essential normal aspect of doing business.
Hedging, unlike arbitrage, is not riskless. What it accomplishes is not the
elimination of risk, but its specialization: its decomposition into its components.
Yes, the remark on the basis is classic and nonetheless easily forgotten nowadays. For a cash position, the basis risk exposure coming out of a future hedge can be huge. In practise, the perfect hedge rarely exists, thus hedging creates an alternative source of risk. It is then essential to start with a correct understanding of the situation. Here, modelling may help in structuring the problem by breaking it down into manageable parts. It aids in identifying key variables, relationships, and constraints involved before taking any decision.
While modeling and analysis are valuable tools, it’s however essential to recognize their limitations. Models are simplifications of reality and may not capture all aspects of a problem accurately. Therefore, it’s crucial to use them judiciously, validate their assumptions, and interpret results with caution. Additionally, some problems may not require formal modeling and analysis, especially when simpler approaches suffice.
This is perhaps the first takeaway. Commodity hedging is primarily being aware of which risks to retain in the portfolio while reducing others. This necessitates a comprehensive understanding achieved through appropriate analysis and modeling.
But is this just a story of modelling, finding the best feasible proxy hedge in time, geography, grade? No, there is also something of hedging culture.
The hedging culture, time- and context-dependant
In oil market, it really depends who you are. For example, the airlines companies have always been known to be frequent users of commodity derivatives. On oil producer side, this says: “While shale’s hedging decisions are a matter of survival or bankruptcy, a very different dynamic is playing out among the oil majors. As their downstream and midstream segments create a natural hedge for their production they do not, by and large, embrace long-term commodity derivatives.“.
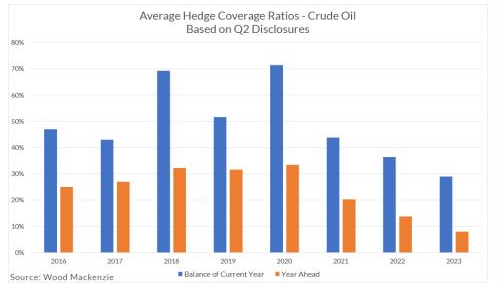
The varying hedging ratio, which tends to be higher in electricity/gas markets than in the oil market, which fluctuates from one year to another, highlights the volatile nature of hedging.
Agriculture market is a place with no golden rule, here: “Many participants in the 2008 survey said they have no actual hedging policies at all or carry out their hedging through a largely subjective process.“, and here:” Moreover, the convention in the industry was to pass on to consumers any rise in costs. Note that some would also focus only on FX and interest rate exposure, keeping open on their commodity exposure.
A second takeaway is that commodity hedging is not a one-size-fits-all policy. The versatile nature of speculation is present, but it is desired and controlled.
The digital transformation impact
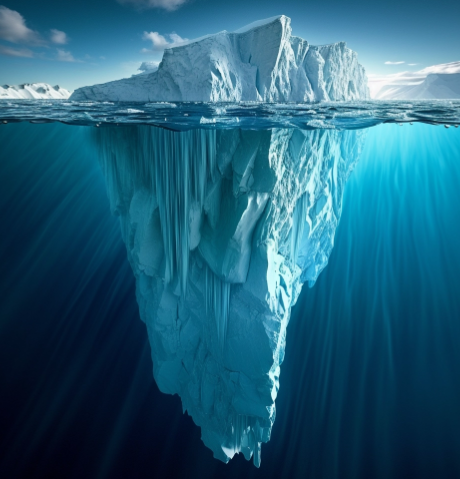
“THE AGE OF data is upon us – and the scale of it is near-incomprehensible. … 370 billion-plus emails and over 100 billion WhatsApp … 500 minutes of video is uploaded … every 60 seconds. And this is just the tip of the iceberg […]
Over time, visualisation and analytics processes will become increasingly automated. … to use artificial intelligence and machine learning to help them find patterns in data … likely to use predictive analytics to prepare for what may change in the market …”
These nice words are from here, and this message has been heard for a quite a long time now. In commodity markets, it often comes with the iconic algorithmic trading. But the article recognizes also that the truth lying behind is very different today: “Most companies understand the value data can provide – however, they may not be set up to properly take advantage of the data that’s available to them.[…] Most of them have grown their use of systems without a plan, leading to a proliferation of data and systems.“.
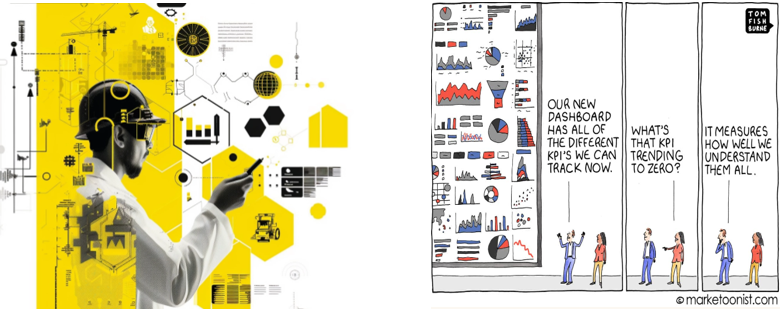
These problems are very well identified now, and are more due to organizational reasons in company more than real technical issues: centralized data models and data team, data silos, slow response time to changing data needs (*), insufficient change management. As read, “companies need to properly understand how to make the most of the data they have in their system“. I would add “that’s far from an easy task”. It involved deep questions and changes in companies’ organisation, roles, and culture. This takes time to evolve. Paradoxically, this search for making critical decision faster sheds light on the need for doing very slow changes in businesses, companies are made of people rather than bits.
The digital age – with its fantasm of predictive modelling – has probably opened the door to more confusion. The fraternal twins may be just reinventing themselves through algoritm’s testing and biases. Note that I believe there is definitively space for improved market prediction in some markets, with accurate data and data treatment (**). But I suspect we easily turn a blind eye in many others, where the lack of critical data and quality is significant, some trying to know for certain a unforeseable future. Before setting predictive modeling objectives, I would take a cautious approach here. I would try to draw first some sort of limit between foreseable and unforeseable components. And then, with adequate management of this data deluge, I sincerely believe that some risks can be better understood and thus properly hedged.
The final takeaway:
From a hedging perspective, the data revolution must be fully embraced. With realism about its true potential and impact in company working culture. With adequate data management, some risks can be better understood and thus properly hedged.
It’s essential to continue acknowledging our biases, questioning our opinions, doing so gradually but steadily
(*): in another article, will talk about a related and interesting concept data mesh.
(**): note that there is certainly success story in term of predictive analytics, such as the european electricty market intraday/day ahead, where relevant variables are easily retrieved (the best weather forecast, the physical asset state in real time, …).